Yichong Wang, Chao Xu, Qianru Lin, Wupeng Xiao*, Bangqin Huang, Wenfang Lu, Nengwang Chen, Jixin Chen*
Ocean and Coastal Management
https://doi.org/10.1016/j.ocecoaman.2024.107250
Published: 01 September 2024
Abstract
Algal blooms represent a pivotal ecological phenomenon in marine ecosystems, exerting significant influence on energy transfer, material cycling, and ecological structure and function. The frequent occurrence of harmful algal blooms (HABs) directly jeopardizes ecosystem integrity, compromises food safety, and challenges the sustainability of food supply, thereby affecting socio-economic development and human well-being. In recent decades, technological advancements in in-situ monitoring and remote sensing have greatly improved our capacity to monitor the oceans, providing unparalleled opportunities for not only the detection of algal blooms but also their dynamic processes and mechanisms. However, monitoring programmes are not always capable of covering the temporal and spatial scale of algal blooms, especially in open water ecosystems. Therefore, it remains essential to utilize models for interpreting observational results. In this review, we evaluated recent studies on models of algal blooms, which can be categorized into three main types: conceptual models, empirical-statistical models (from simple machine learning models to state-of-the-art deep learning models), and mechanistic models (process-based models). We reviewed the development and refinement of representative cases for each model type and compared their applicability, strengths, and limitations. Subsequently, we summarized the applications of these models in exploring algal bloom dynamics, short-term forecasting (a few days to seasonal), and long-term predictions (interannual or longer). We also proposed several recommendations for how algal bloom modeling can move forward: 1) Integrate various automated monitoring platforms and remote sensing techniques to obtain more comprehensive data for model validation; 2) Develop more powerful deep learning models to fully exploit their immense potential in algal bloom prediction; and 3) Incorporate molecular ecology and omics technologies to enhance parameterization schemes for numerical models.
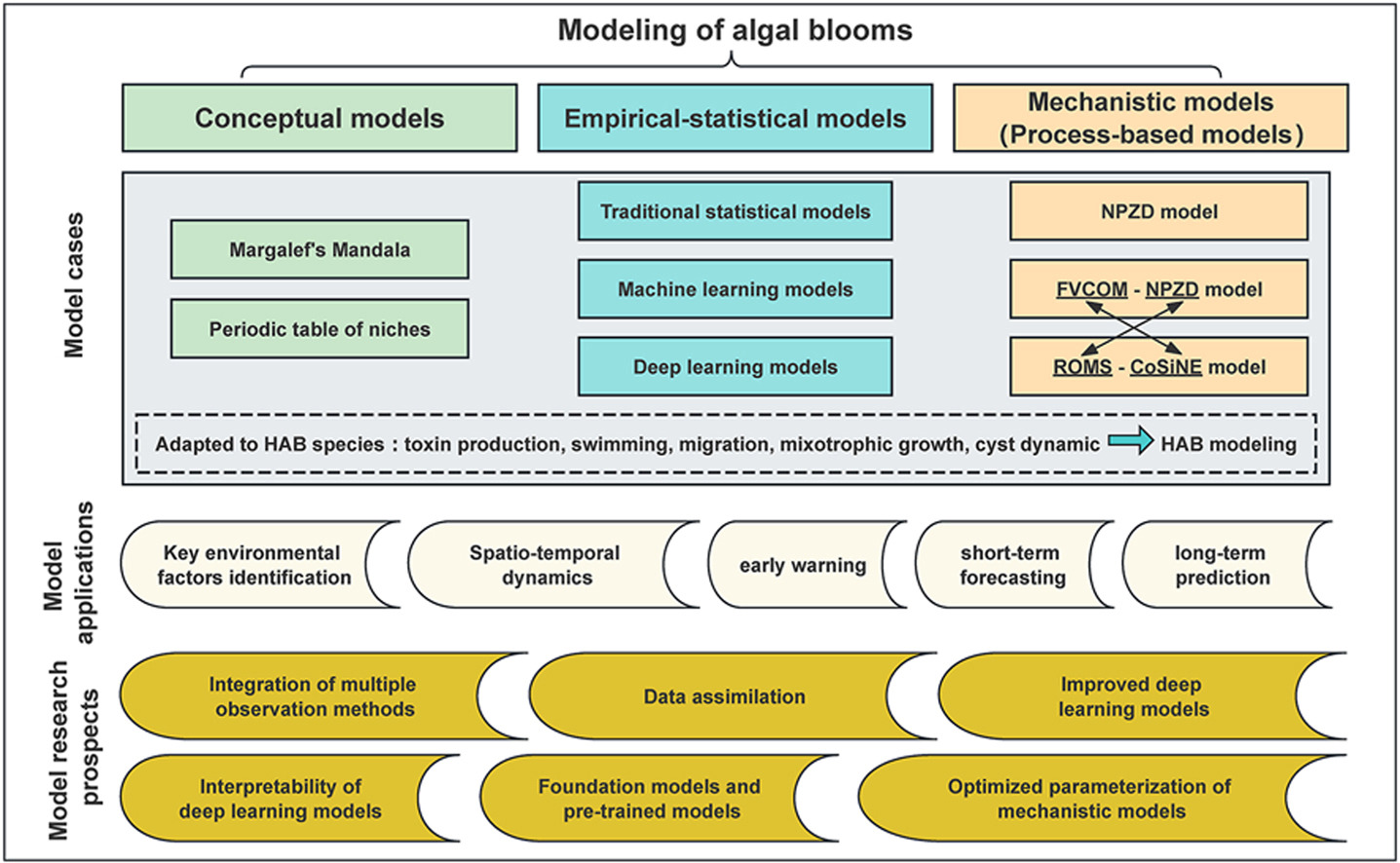